This report is part of our Reshaping Supply Chains for the 2020s series, in which we analyze the technologies and trends that are enabling the evolution of the supply chain.
Retailers are increasingly recognizing the importance of demand forecasting. More accurate forecasts can help to minimize inventory costs, optimize distribution and merchandising, and streamline the selling process. In this report, we highlight key trends in demand forecasting and offer examples of key innovators and retail success stories.
The supply chain comprises seven major components that are integral to a retailer’s business model and can represent key sources of competitive advantage and differentiation: Companies that get their products to market faster and more efficiently than their competitors can better harness the opportunity to make a positive impact in the market. The execution of component processes is supported by various technologies throughout the supply chain—from product design to end-consumer.
[caption id="attachment_112241" align="aligncenter" width="700"]
Source: Coresight Research[/caption]
Improving Demand Forecasting Has Become a Key Initiative for Retail Leaders
Forecasting is difficult, especially when it comes to anticipating consumer demand for new products and determining sales volumes and promotions, as these processes include billions of data points. Many retailers still work with spreadsheets or use classical time-series-based solutions to forecast the future. However, manually collecting information about influencing factors is time-consuming, and the results of correlated data can be poor.
Some 42% of US retail executives said demand forecasting is the largest pain point that they need to resolve, and 46% of them wish to have robust technology, according to a Coresight Research poll of 80 senior-level retail executives conducted in March 2020. Improving demand forecasting has thus become a key initiative for retail leaders.
Demand forecasting is the process in which both passive and active data are used to develop an estimate of future consumer demand, which is applied to operational procedures and strategic decision-making to maximize sales and profits. Over 50% of organizations believe that advanced sales and operations planning (otherwise known as integrated business planning) is the top key business process to which demand forecasting will contribute by the year 2025, according to a recent survey from the Journal of Business Forecasting.
Other applications of demand forecasting in the supply chain include the following:
- Collaborative planning and forecasting replenishment—A business practice that combines the intelligence of multiple trading partners in the planning and fulfillment of customer demand, such as linking category management to supply chain planning and execution processes to increase availability while reducing inventory transportation and logistics costs.
- Business efficiency planning—A process that uses tools to understand what decisions need to be made, when and by whom, as well as the implications of these decisions for other business operations.
- Product lifecycle management—A process that manages the lifecycle of a product from inception, through engineering design and manufacture, to service and disposal.
- Event-based or marketing-driven sales and operational planning—This includes sensing market conditions based on demand signals, then shaping demand using programming such as price optimization, trade promotion planning, new-product-launch plan alignment and social or digital adjustment.
Machine Learning Is a Core Competency in Demand Forecasting
Only 9% of the global supply chain professionals surveyed by AIMMS (a prescriptive-analytics software company based in Europe) in September 2019 used ML, but 40% are actively investigating this technology (see Figure 2). We expect more retailers to replace their current techniques with ML or add relevant capabilities to their current demand forecasting solutions over the next three years.
[caption id="attachment_112242" align="aligncenter" width="700"]
Base: 200+ global supply chain professionals, surveyed in 2019Source: AIMMS[/caption]
ML can leverage both structured and unstructured data to generate complex mathematical algorithms that recognize data patterns and capture demand signals. These capabilities enable ML-based solutions, such as predictive analytics, to produce more accurate forecasts in complex scenarios.
A 2019 survey of 114 global retail executives by Coresight Research found that 56% of respondents used predictive analytics technology and 45% of respondents planned to invest in it in the following 12 months—making it one of the survey’s highest-ranked technologies in terms of usage and investment. Predictive analytics generates intelligence based on data regarding historical business performance and relevant external factors, such as weather, economic indicators, calendar events and seasonality. Retailers are turning to predictive analytics to help solve difficult problems in demand forecasting and uncover new opportunities.
Below, we outline some recent examples of retail companies partnering with supply chain solutions providers to apply demand-forecasting techniques:
- Sales forecasting and staff planning—Germany’s largest drugstore chain DM partnered with supply chain solutions company Blue Yonder to predict sales accurately in its individual stores and thus carry out reliable staff planning. In addition to each store’s daily sales from the last 10 years, the forecasts considered adjustable parameters such as store hours, seasonality, location, events and prices.
[caption id="attachment_112243" align="aligncenter" width="700"]
Blue Yonder’s ML-based Luminate Planning solution considers adjustable parameters such as seasonality and locationSource: Blue Yonder[/caption]
- Inventory management—Belk, an American department-store chain, used Antuit.ai’s demand-forecasting solutions to generate an accurate enterprise demand signal that drives improved planning and fulfillment at the SKU (stock-keeping unit) level across the entire organization. The retailer’s previous forecasting practices did not incorporate statistical modeling, and replenishment plans were based on the average rate of historical sales rather than future predictions. The forecast now takes into account seasonality, promotions, events and other relevant variables to arrive at a stable and accurate forecast.
- Merchandise planning—Soludos, a footwear company founded in 2010, offers hundreds of SKUs across multiple categories and solved pain points in merchandising planning by working with Alloy, an analytics platform. Alloy helped the brand to collect and understand point-of-sale (POS) and inventory data across direct-to-consumer, e-commerce and retail operations to analyze product performance and identify merchandise that sells well, by features such as style, size and color.
- Markdown optimization—Family Dollar successfully replaced its enterprise-wide markdown strategy with a localized markdown plan by partnering with Revionics, a startup that offers lifecycle price-, promotion- and markdown-optimization solutions for the retail industry. Revionics’ predictive science supports replenished merchandise and category-management processes, and it can forecast markdowns that could improve profit margins. Following the partnership, Family Dollar has seen 10% higher sell-through for categories under testing.
- Marketing—RaceTrac, which operates a chain of gasoline service stations across the Southern US, partnered with business intelligence solutions provider Prevedere to understand the expected future outcome of advertising campaigns and improve forecasts of guest counts, margins and labor expenses. Prevedere’s correlation engine identifies relevant indicators from among millions of data series using the computing power of the cloud, and its patented genetic algorithm generates the optimal forecast model for the business.
[caption id="attachment_112244" align="aligncenter" width="700"]
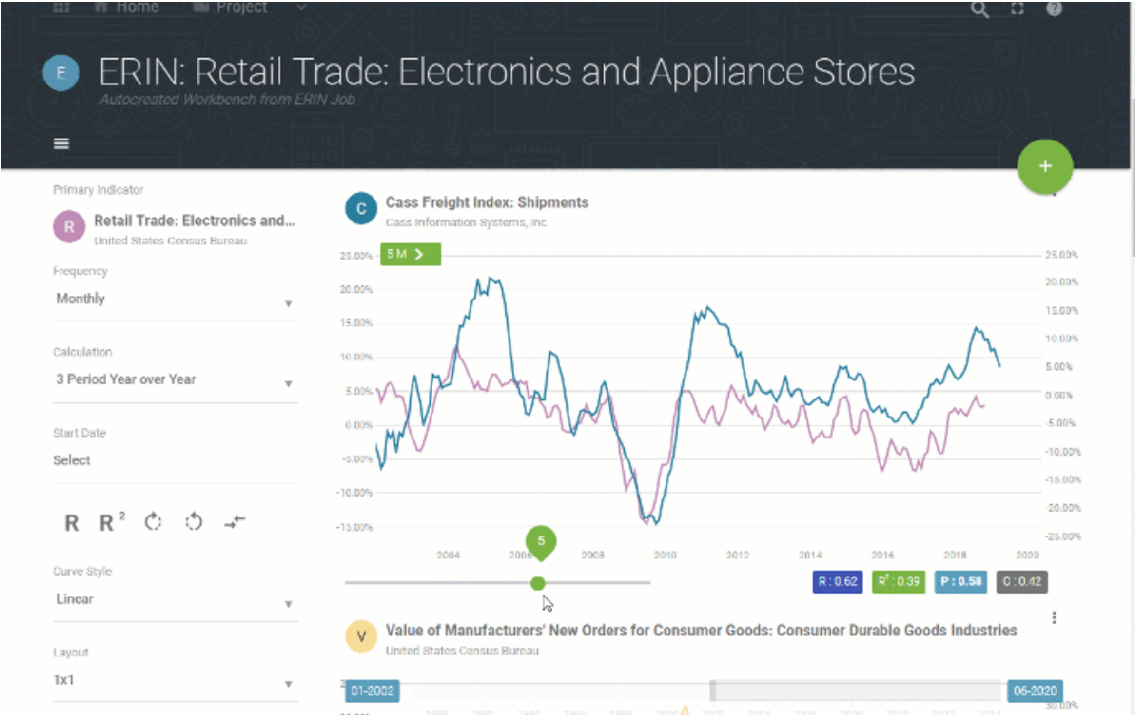
Prevedere’s ERIN (External Real-time Insights) Engine allows users to upload historical data (monthly product sales, revenue and category performance) to generate predictions for a particular industry or geography.
Source: Prevedere[/caption]
Businesses Are Exploring Other Techniques Besides Machine Learning
Besides machine learning, businesses are exploring other demand-forecasting techniques, such as statistical modeling of historical demand (which is more traditional and includes time-series modeling) and multi-level forecast hierarchies (coordinating separate models for different hierarchy levels so that forecasts are consistent and accuracy is improved).
Demand sensing is another technology that is gaining traction in retail. It captures real-time data from POS systems, warehouses and external sources, and offers recommendations and adjustments. Demand sensing is typically used for two purposes:
- Inventory management—Philip Morris International, a tobacco company, partnered with supply chain software provider E2open to generate accurate near-term forecasts regarding inventory. The solution uses real-time data, automation and artificial intelligence (AI) algorithms, and enables the retailer to improve fulfillment and replenishment by having visibility over stock.
- Sales forecasting—Amer Sports oversees some of the world’s leading sporting goods brands, including Arc’teryx, Atomic, Mavic, Salomon, Suunto and Wilson. The company worked with planning software provider Anaplan to set realistic sales targets and determine consistent rewards for its staff across different brands and regions. Anaplan performs scenario analysis in real time.
In addition, DDMRP is an alternative solution to improve forecasting and plan inventories and materials. A good example of the use of DDMRP in retail is PZ Cussons, a major British manufacturer of personal care products and other consumer goods. The retailer operates in 14 countries covering Africa, Asia and Europe. It partnered with O8, a DDMRP solution provider that supports the management of supply chain planning and operational processes, to establish a model for supplying goods from one country to another and reducing UK involvement in the planning of its Thai supply chain. The resulting holistic and standardized business planning solution realized a 25–30% reduction in inventory costs for PZ Cussons.
What Does the Future of Demand Forecasting Look Like?
- Highly automated: More retailers and brands will focus on ML and AI as the core technologies underlying demand forecasting solutions, as these can realize a high degree of automation—applying algorithms with minimum intervention from data scientists. Some innovators have already begun to disrupt this sector. For example, in the last three years, Blue Yonder has built ML within a framework called cyclic boosting. The framework allows users to have transparency over demand forecasting and the automated decision-making behind it—which is applicable to grocery retail operations, for example.
- More granular and in real time: The future of demand forecasting will not only be based on historical data but also on real-time information as it is streamed from connected devices. Retailers will strive to gain more granular data, taking into account all available data—such as weather, store opening hours, in-store behavior, shopping baskets, POS, events and SKU-level data—in a dynamic and fast-adapting forecast solution. Real-time data is particularly important for fresh and perishable goods sectors because they require low-latency forecasts due to continuously shifting product supply and consumer demand.
- Cross-functional: Demand forecasting will adopt a cross-functional role instead of representing a silo in the supply chain. It is increasingly important for retailers to have a holistic strategy to improve the accuracy of demand forecasting, comprising enterprise data, applications of advanced forecasting methods and synchronized cross-functional business processes. Demand forecasting could also be incorporated into financial reporting and analysis, enterprise risk management and human resource planning.
- Input-driven: Data quality is a critical component of accurate demand forecasting. Instead of managing the output, future demand planners will be managing the input: High-quality data will lead automated solutions to generate the most accurate forecasts.
Key Insights
Demand forecasting is the cornerstone for the various stages of the retail supply chain. By improving this capability, companies can anticipate consumer needs and preferences and adapt their marketing and promotional campaigns accordingly, as well as incorporating such intelligence into pricing strategies and product assortment. We expect vendors with ML-based solutions for demand forecasting (such as predictive analytics) to lead disruption in this sector. The future of demand forecasting will be highly automated, more granular and in real time, cross-functional and input-driven.